How to Attract the Girls: Gender-specific Performance and Motivation to learn Computer Science
In this blog post, Peter Hubwieser summarizes his work (with co-authors Elena Hubwieser and Dorothea Graswald) that was published in a 2016 journal article. Here, he highlights research exploring the importance of reaching girls earlier through motivation.
The attempt to engage more women in Computer Science (CS) has turned out to be a substantial challenge over many years in many countries. Due to the obvious urgency of this problem, over the last decades many projects have been launched to motivate women to engage in Computer Science. Yet, as already very young girls seem to have different attitudes toward CS compared to even-aged boys, all attempts to influence adult women might come too late.
Potentially, the international Bebras Challenge could provide a facility to arouse the enthusiasm of girls for Computer Science, aiming to promote Informatics and Computational Thinking. During the most recent event, Bebras attracted nearly 3 Million participants from 54 countries, separated in different age groups.
As shown e.g. by Deci and Ryan in 1985, motivation is likely to correlate with the personal experience of competency. Although most participants of Bebras are encouraged to participate by their teachers, they solve the tasks individually or in pairs. Therefore, the individual motivation of the students might play a dominant role in their performance. In addition, the Bebras challenge will have a positive impact on the children only if they are able to solve a satisfying number of tasks.
To find out if this is the case and to detect differences between boys and girls, we analyzed the outcomes of the 2014 challenge regarding the gender of the 217,604 registered participants in Germany. Additionally, we compared the average performance of boys and girls in every task.
The boys were more successful overall, and the differences increased dramatically with age. Nevertheless, it turned out that in the two younger age groups (grade 5-6 and 7-8 respectively), girls outperformed boys in several tasks. The analysis of these tasks demonstrated that in particular, girls can be motivated by the first three factors of the ARCS Model model of motivation (see Keller 1983): Attention, Relevance and Confidence.
After measuring the performance, we grouped the 27 tasks according to the difference in performance of boys and girls:
- Girls’ Tasks: 7 tasks that were solved significantly better by single and paired girls,
- Boys’ Tasks: 13 tasks that were solved significantly better by single and paired boys,
- Neutral Tasks: 7 tasks, either without any significant gender difference or showing such a difference in only one case (singles or pairs).
Assuming that each task needs to attract attention, its first impression is likely to be crucial. Therefore, its graphical elements like pictures or diagrams are highly relevant. Looking at the graphical elements of the Girls’ Tasks, we found that these were mostly representing animals, jewelry or food (see Fig. 1). Regarding the Boys’ tasks, the dominating elements were mostly abstract rectangular figures, graphs or technical apparel. The Neutral Tasks had an appearance that is more or less similar to the Boys Tasks (see Fig. 2).
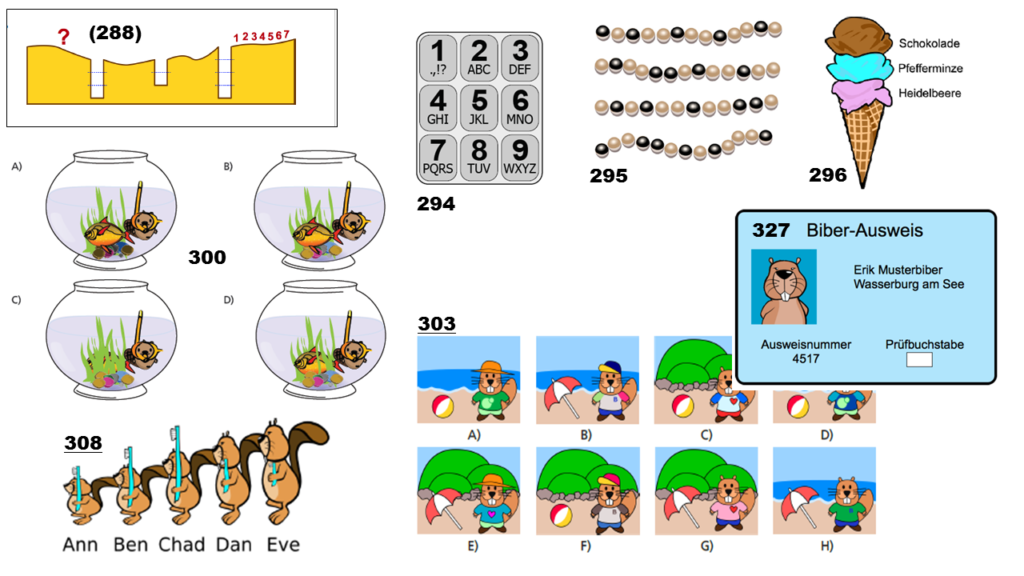
Figure 1. Girls’ Tasks Pictures
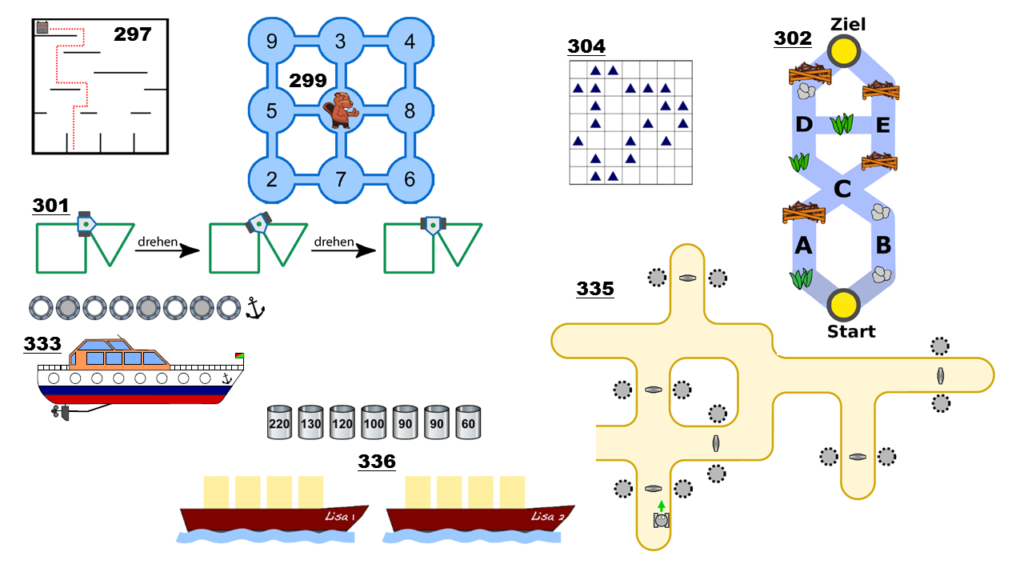
Figure 2. Boys’ Tasks Pictures
In this context, relevance is likely to be determined by the probability that the participants or their friends have experienced or will experience a similar situation. Obviously, all of the Girls Tasks showed a certain relevance, e.g. how to identify your own bracelet. On the other hand, all of the Boys Tasks lacked this relevance at least for girls, e.g. how can a robot cross a labyrinth. Also the Neutral Tasks missed relevance more or less, except a few that didn’t look attractive or were too difficult (see below).
Third, the apparent difficulty (as assessed by the participants) of a task will influence the motivation or the confidence to solve it. Indeed we found that the girls tended to perform significantly better than the boys in tasks of low or medium difficulty. The explanation might be that the self-efficacy of girls was lower compared to the boys. On the other hand, the boys seemed to show higher willingness to deal with challenging problem solving activities by trial and error.
Yet, we should keep in mind that these results emerged in a contest, where the decision to work on a certain task was voluntary, in contrary to compulsory assignments in the classroom. Nevertheless, CS educators might use these findings to construct tasks that motivate particularly younger girls:
- Look for a situation that is likely to occur in girls’ everyday life,
- Construct a task for this situation that is not too difficult, and
- Draw a nice picture that contains a person, an animal or other lovely objects to attract attention.
Peter Hubwieser, Technical University of Munich (Germany), taught math, physics and computer science at high schools until 2001. In 1995 he completed his doctoral studies in physics. In 2000 he acquired his postdoctoral teaching qualification (habilitation). In 2002 he was appointed to a professorship position at TUM. He has worked as visiting professor in Austria (Klagenfurt, Salzburg and Innsbruck), France (ENS in Paris and Rennes) and Michigan (MSU). His research activities focus on the empirical investigation of learning processes in computer science. His novel didactical approach triggered the introduction of computer science as a compulsory subject at Bavarian Gymnasiums in 2004.
Elena Hubwieser and Dorothee Graswald completed their teacher education at TUM, where they have conducted this research in collaboration with Peter Hubwieser. Currently they are teaching math and computer science at Bavarian Gymnasiums.
The original paper was published in a Springer Proceedings Volume: Hubwieser, P., Hubwieser, E., & Graswald, D. (2016). How to Attract the Girls: Gender-Specific Performance and Motivation in the Bebras Challenge. In A. Brodnik & F. Tort (Eds.), Informatics in Schools: Improvement of Informatics Knowledge and Perception: 9th International Conference on Informatics in Schools: Situation, Evolution, and Perspectives, ISSEP 2016, Münster, Germany, October 13-15, 2016, Proceedings (pp. 40–52). Cham: Springer International Publishing. https://doi.org/10.1007/978-3-319-46747-4_4
For more CS education insights, view our blog.